Welcome Professor Shahzad!
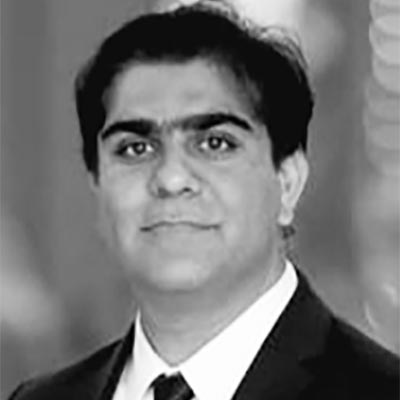
We are happy to share that now we count on the presence of Asst. Prof. Dr.-Ing. Muhammad Shahzad as Guest Professor at the AI4EO Future Lab, performing as co-leader of the Uncertainties lab key topic.
Professor Shahzad studied Electrical Engineering at the National University of Sciences and Technology (NUST) of Pakistan. He holds a Master´s degree in Autonomous Systems from the Bonn-Rhein-Sieg University of Applied Science, in Sankt Agustin, Germany. His Engineering Doctorate was granted in February 2016 by the Technical University of Munich, for his work in Remote Sensing and 3D Computer Vision. Afterwards, he has worked as Assistant Professor at NUST where he served as co-director of the Machine Vision and Learning Lab at NUST and deep learning lab at the National Center of Artificial Intelligence. Besides NUST, he possesses research experience in serving various prestigious institutions including German Aerospace Center in Oberpfaffenhofen, the Technical University of Graz in Austria, and the Fraunhofer Institute for Intelligent Analysis and Information Systems in Sankt Augustin.
The focus of his research is the development and application of AI algorithms, in particular deep learning, to address remote sensing and 2D/3D computer vision problems.
We share next a brief interview done to Professor Shahzad:
Thank you Professor Shahzad for taking the time for this conversation:
1. Could you please tell us what have been your latest research topics and interests?
From application perspective, I have been focusing on diverse range of topics lying within the domains of optical/radar-based satellite (e.g., deforestation mapping, land cover mapping, crop-type classification & object reconstruction), and airborne (e.g., crop health and height estimation, vision-based 3D localization of drones, semantic segmentation of 3D point clouds, & object detection) remote sensing. Apart from these, I also gained experience in processing natural images using advanced deep learning techniques to address core computer vision problems including object (person/vehicle) re-identification, image matching and dense image captioning.
Recently, I have been intrigued by the idea of self-supervised learning algorithms that aim to exploit the unlabeled data to finetune pretrained (supervised) networks for several pretext tasks. The visual representation learned by these self-supervised algorithms has shown remarkable progress in the last couple of years and is consistently reducing the performance gap when compared with their counter supervised approaches on large-scale computer vision benchmarks.
2. You have joined the lab group that is working with Uncertainties. What do you think are the biggest current challenges of this topic?
It is really fascinating to see how deep neural networks can be so effectively used to tackle wide range of applications by employing the core idea of feature learning. However, they are still somewhat mysterious in the sense that it is often difficult to explain the decision process of the trained network which consequently raises the question about its certainty in the prediction. Therefore, in my opinion, the core challenges of this topic are twofold: First, the lack of ground-truth uncertainties really makes it difficult to precisely quantify and validate the available uncertainty estimation methods. Secondly, it is hard to infer the actual reasons for which the network confidently but falsely makes the prediction. The “explainability” factor is not yet reached to a level with which we can confidently assess the cause of uncertainty with our current understanding of the field.
3. What would be in your opinion the trends of 3D modelling based on satellite data? What are the current needs and applications that could be covered with such development, and what is the advantage of this methods?
3D modeling based on satellite data is a vital component in developing smart cities. For instance, having a 3D model of the city can open up several application domains, e.g., estimating the insolation of buildings by gauging how much a building is exposed to sun, calculating the energy demand of households, classifying building types, performing urban visibility analysis, assessing shadow casting, noise propagation, planning urban developments, deploying communication infrastructure and many others. Currently, these 3D models are generated using semi-automated methods employing LiDAR and/or photogrammetric techniques to extract the 3D information and are only available in the developed countries. The reason being that most of the available datasets only include instances of objects appearing in these demographic regions which limits their generalization ability to cope with the varying structures and appearances of the man-made structures in the other parts of the globe (e.g., developing and under developed countries).
4. Which impact on the scientific community would you like or expect that your work and that of your topic team have by the end of the AI4EO Future lab project?
I am really excited to see many different researchers with diverse backgrounds, but with mostly similar interests have joined hands and are working together. I am hopeful that this would pave way to valuable scientific insights and research findings as the considered topics go beyond the general trends in machine learning and earth observation. At the end, I would be happy if we are able to explore and quantify the uncertainties pertinent to often loosely annotated remote sensing datasets and further use them to gain valuable insights from network’s explainability perspective.
5. In your opinion what is the biggest challenge of artificial intelligence nowadays?
In my opinion, explainability and quantifying uncertainties in the AI predictions is the foremost challenge since the usage of AI is trending towards applications which are of critical nature and require trustworthy AI architectures.
6. Would you share with us what are your hobbies? How do you enjoy your free time?
Usually, I spend free time by doing some physical activity e.g., running, walking or biking.
7. You already had lived in Munich. What are in your opinion the most enjoyable things of this city and its surroundings?
Munich is beautiful. I like the atmosphere here. The city is vibrant and is a cultural hub which really fascinates me about it.
8. Which is your home city? What is the most enjoyable thing from there to you?
I live in Islamabad which is the capital of Pakistan. The most fascinating thing about Islamabad is its geolocation. It lies at the foothill of the beautiful Margalla Hills, so you can drive up to an hour towards north to see snow in winter on tall mountains. On the other hand, you can drive one hour towards south to see gorgeous plains of the Punjab region.
Thank you for sharing with us your perspectives and a brushstroke about yourself. The AI4EO Lab team welcomes you and is very looking forward to coming joint work.